FROM:
Eur J Phys Rehabil Med 2024 (Feb); 60 (1): 145–153
~ FULL TEXT
Participants in the 5th Cochrane Rehabilitation Methodological Meeting:
Pierre Côté • Stefano Negrini • Sabrina Donzelli • Carlotte Kiekens • Chiara Arienti
Maria G Ceravolo • Douglas P Gross • Irene Battel • Giorgio Ferriero
Stefano G Lazzarini • Bernard Dan • Heather M Shearer • Jessica J Wong
Institute for Disability and Rehabilitation Research,
Faculty of Health Sciences,
Ontario Tech University,
Oshawa, ON, Canada.
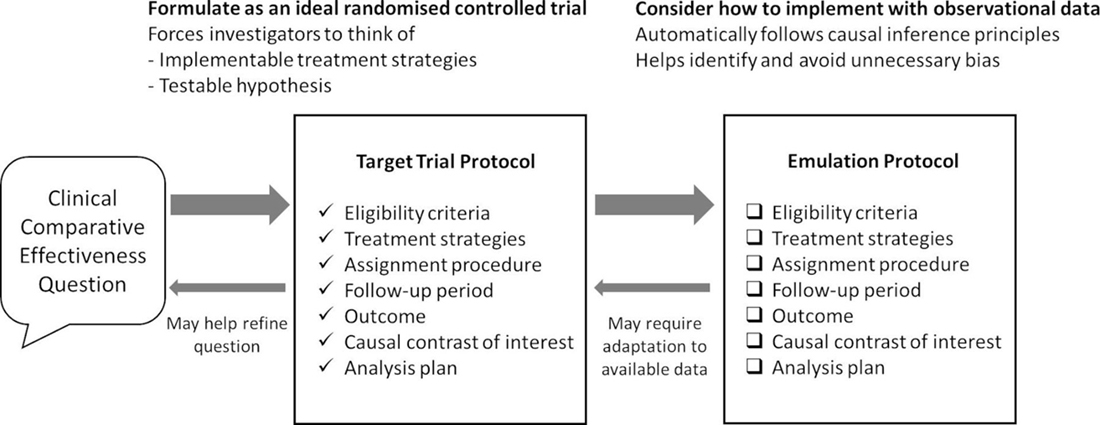
Target Trial Emulation Strategy
Rehabilitation providers and policymakers need valid evidence to make informed decisions about the healthcare needs of the population. Whenever possible, these decisions should be informed by randomized controlled trials (RCTs). However, there are circumstances when evidence needs to be generated rapidly, or when RCTs are not ethical or feasible. These situations apply to studying the effects of complex interventions, including rehabilitation as defined by Cochrane Rehabilitation. Therefore, we explore using the target trial emulation framework by Hernán and colleagues to obtain valid estimates of the causal effects of rehabilitation when RCTs cannot be conducted. Target trial emulation is a framework guiding the design and analysis of non-randomized comparative effectiveness studies using observational data, by emulating a hypothetical RCT. In the context of rehabilitation, we outline steps for applying the target trial emulation framework using real world data, highlighting methodological considerations, limitations, potential mitigating strategies, and causal inference and counterfactual theory as foundational principles to estimating causal effects. Overall, we aim to strengthen methodological approaches used to estimate causal effects of rehabilitation when RCTs cannot be conducted.
Key words: Rehabilitation, Comparative effectiveness research, Randomized controlled trial, Cohort studies, Observational study
From the FULL TEXT Article:
Background
Rehabilitation providers need quality evidence to make timely clinical decisions. Similarly, policymakers require valid research to plan and implement healthcare delivery models that address emerging rehabilitation needs in the population. In an ideal world, these decisions should be informed by randomized controlled trials (RCTs). However, in circumstances where RCTs are not warranted or possible (e.g., when effectiveness data is urgently needed, RCTs are not ethical or feasible for various reasons including costs), best practices or observational studies are often used to inform clinical decisions. For example, the delivery of rehabilitation to patients with moderate to severe COVID-19 during the early phases of the pandemic [1–3] or the field of stroke rehabilitation [4] are largely informed by best practices and observational studies. Therefore, it is necessary to consider how alternative designs to RCTs can provide valid estimates of the cause-and-effect relationship between an intervention for rehabilitation and functioning.
Target trial emulation is a framework used to guide the design and analysis of comparative effectiveness (non-randomized) studies using “real world” observational data that emulates a hypothetical RCT. In this article, we explore how applying the target trial emulation framework by Miguel Hernán and colleagues can provide valid estimates of the causal effects of rehabilitation when RCTs cannot be conducted. [5, 6] Furthermore, we outline the methodology used to design cohort studies and quasi-experimental studies based on target trial emulation, including trial protocol development and the use of observational data necessary to make causal inferences. As illustrated below, our discussion focuses on investigating the comparative effectiveness of rehabilitation grounded in causal inference and extends the principles of benchmarking-controlled trials proposed by Malmivaara. [7]
Ethics, scientific justification, feasibility,
and timeliness of RCTs: a balancing act
It is indisputable that RCTs are the design of choice to answer causal questions about the effectiveness of an intervention, such as rehabilitation. The methodological advantage of RCTs comes from the ability to control for confounding through randomization.
However, randomization is indicated under specific circumstances:
1) when clinical equipoise is present; [8]
2) when the interventions being tested do not exceed accepted minimal risks;
3) when it is acceptable to patients; or
4) previous research justifies its conduct. [9]
Establishing clinical equipoise is necessary yet challenging and should be demonstrated by conducting a systematic review of the literature, conducting qualitative research or surveying experts, health care providers and patients, and by understanding patient preferences.10 The ethical conduct of an RCT also requires that the interventions being tested do not exceed accepted minimal risks. For example, it would be unethical to estimate the effects of high-dose opioids on pain intensity in children with cerebral palsy because of the high risk of addiction and other side effects.
There are also situations when conducting an RCT is not feasible because evidence is urgently needed to make clinical timely decisions (e.g., rehabilitation for patients in emergency situations), the costs of conducting an RCT can be prohibitive (e.g., robotic rehabilitation combined with brain-computer interface technology for patients with spinal cord injury) and recruiting enough participants may not be possible because a disease is rare. In rehabilitation, RCTs are often not feasible as interventions in rehabilitation have small effects and the disease may be rare, posing challenges with sample size and reliability. Nonetheless, evidence is strongly needed and strategies with alternative study designs can overcome these challenges. Finally, as described by Shearer et al.,9 testing the effectiveness of rehabilitation in an RCT requires that preliminary research supports its feasibility, potential benefits, and safety for patients.
Rehabilitation and comparative effectiveness
According to Cochrane Rehabilitation, rehabilitation includes a multimodal, person-centered, and collaborative process, that targets a person’s capacity or contextual factors related to performance with the goal of optimizing functioning. [9, 11] Rehabilitation is inherently complex and needs to be tailored to an individual’s needs. The complexity of rehabilitation resides in its structure, delivery mode and targeted outcomes, while its design requires combining clinical interventions and technologies, clinicians, settings, and recipients of the intervention (patients, family, and community). Therefore, applying the target trial emulation framework to rehabilitation necessitates careful consideration of the complexity of the experimental and control interventions.
Since rehabilitation needs to be tailored to the specific needs of study participants, it is necessary that the sources of data used to emulate a trial describe the ingredients of the rehabilitation program in sufficient detail for clinicians to understand what and how it was tailored to patients’ individual needs. This description requires that the data about the intervention are sufficiently detailed to differentiate the experimental from the control group and allow the valid classification of patients into the correct intervention group.
Table 1
|
It is useful to consider the emulated target trial by Heil et al. to illustrate how rehabilitation compared within a target trial intervention framework should be described. [12] The authors aimed to determine the effectiveness of a multimodal prehabilitation program compared with usual care in high-risk patients with colorectal cancer who underwent elective colorectal surgery. The detailed treatment strategies (multimodal prehabilitation versus usual care) as described by Heil et al. [12] are outlined in Table I.
Heil et al. provided adequate details of the intervention and comparison groups, including all components and provider details of the multimodal prehabilitation program and usual care, respectively. [12] These details were captured in the data source they used and allowed for correct classification of patients into the two groups when emulating a target trial.
Causal inference
Making valid causal inferences requires that we use counterfactual theory. [13–15] In an ideal situation, we would compare the potential outcomes for the same individual under two contrasting conditions (e.g., treatment A versus treatment B) for causal inference. However, one of these conditions is real (factual) and the outcome can be observed, while the other condition is counterfactual and the outcome cannot be observed (termed ‘counterfactual’ or ‘potential outcome’). Let us apply counterfactual theory to studying whether a vocational counselling intervention is associated with return to work in patients with rheumatoid arthritis. To determine whether vocational counselling improved the return-to-work rate requires that we answer the question “Would the patients have returned to work at the same time (outcome) had they not received vocational counselling?”. In an ideal situation, we would be able to observe the outcome for the same patients at the same time but treated with usual care instead of vocational counselling. Of course, this is not possible, and we cannot make this observation; it is counterfactual. Since observing the outcome in the same individuals at the same time under two distinct intervention scenarios is impossible, we must rely on comparative experiments to estimate the effect of an intervention.
To make causal inferences using counterfactual theory, a study must meet the exchangeability, or “no confounding and no selection bias” assumption. Exchangeability implies that the groups being compared (exposed and unexposed) are similar in all aspects that are of importance to the outcome, except for the exposure. [16, 17] Achieving exchangeability is straightforward in randomized controlled trials but more challenging in observational studies. In observational studies, achieving exchangeability hinges on adequately controlling for confounding, including unmeasured factors through design (e.g., self-controlled studies, Directed Acyclic Graphs (DAGs) and negative control exposures) or analysis (e.g., instrumental variables or model-based adjustment for confounders). [18, 19]
Applying the target trial emulation framework to
quantify the effect of rehabilitation
As described earlier, it is impossible to observe the outcome in the same individual at the same time under two contrasting treatment conditions. To overcome this fundamental problem, we can move to estimating population causal effects, which involves comparing outcomes under different conditions between comparable groups. Tied to the previous example, to quantify the effect of vocational counselling (as an example of rehabilitation) on return to work in patients with rheumatoid arthritis, the rate of return to work can be compared between groups of patients who received vocational counselling to those who did not receive vocational counselling (but received usual care instead). Ideally, these patients with rheumatoid arthritis would be randomly assigned to the two groups to ensure that they are comparable (exchangeable) at baseline. Therefore, any differences in return-to-work rates can be attributed to vocational counselling as opposed to prognostic differences between the two groups. RCTs also provide the advantage of clearly specifying time zero for each participant (time of randomization to the assigned treatment group), which is important for causal inference.
Table 2
|
If an RCT cannot be conducted due to feasibility, ethical issues, or time/resource constraints, observational studies can be designed to incorporate these key features of an RCT for causal inference. Applying the target trial emulation framework, developed by Hernan and colleagues, facilitates this by explicitly emulating a hypothetical RCT that is designed to answer the causal question at hand, which is known as the ‘target trial’. [5, 6, 20, 21] Suppose we would like to design a study to determine the effect of dedicated units of inpatient stroke rehabilitation (a specific model of service delivery in Ontario, Canada) on the risk of fractures in adults with a diagnosis of stroke or transient ischemic attack (TIA). Regarding this specific model of service delivery, dedicated units for inpatient stroke rehabilitation are units in rehabilitation facilities with beds and therapists dedicated to patients with stroke (Table II). [22]
There are potential challenges to conducting an RCT comparing dedicated versus non-dedicated units for inpatient stroke rehabilitation, as these units represent complex interventions rolled out to different facilities at different times, and an RCT with a 2–year follow-up to assess the risk of stroke would be time- and resource-intensive. If we are unable to conduct an RCT to answer this causal question, we can consider emulating a target trial with analyses of observational data. Target trial emulation involves two steps:
Step 1: Articulating the causal question and protocol
Table 3
|
The causal question and protocol would be formulated as though we were designing a hypothetical RCT. This causal question would be the question we ask if we could design an RCT e.g., What is the effect of inpatient stroke rehabilitation delivered through dedicated facility units compared to inpatient stroke rehabilitation delivered through non-dedicated stroke rehabilitation units on risk of fracture (within 2 years post-stroke) in older adults with a diagnosis of stroke? (Table II). The protocol outlines key elements (termed “causal estimands”) of a randomized trial representing the target study to answer this causal question. This includes eligibility criteria, treatment strategies, treatment assignment, start and end of follow-up, outcomes, causal contrasts, and analysis plan (Table III). [5, 6]
Target trial protocol for our example
Using the example of inpatient stroke rehabilitation, we would start by designing a comparative effectiveness study with clear eligibility criteria for the sample (component #1), e.g., older adults (aged ≥65 years) with a diagnosis of stroke or TIA, with specific inclusion and exclusion criteria listed in Table III. Component #2 describes the treatment strategies. For example, this can be dedicated units versus non-dedicated units of inpatient stroke rehabilitation. [22] Component #3 describes how participants are assigned to a treatment strategy in this hypothetical RCT, e.g., randomly assigned to dedicated versus non-dedicated stroke rehabilitation units at baseline in this target RCT. Component #4 describes the follow-up period from baseline (time zero, starts at randomization) to the outcome or end of follow-up, such as ending at the outcome (fracture), death, loss to follow-up, or 2 years after baseline. Component #5 states the outcome of interest, e.g., risk of fracture within 2 years after baseline (i.e., after index date of stroke or TIA). Component #6 refers to causal contrasts such as intention-to-treat effect or per-protocol effect. Lastly, component #7 describes the analysis plan, which would emulate the analysis intended for the target trial, such as to estimate the intention-to-treat or per-protocol effect.
Step 2: Execution of target trial emulation –
Emulating the components of a protocol representing the target trial
The protocol components representing a target trial (hypothetical randomized trial) would be emulated using observational data (Table III). This hypothetical randomized trial represents the target study for causal inference. This step involves adapting the target trial protocol to using observational data, which includes finding eligible individuals, assigning eligible individuals to a treatment strategy based on what is documented in the observational data, following individuals from time zero (time of treatment assignment) up to the outcome or end of follow-up, and developing an analytic plan aligned with the target trial with the addition of adjusting for baseline confounders to emulate randomization. To guide the study design and analysis, directed acyclic graphs (DAGs) are used to represent relationships between variables, including the exposure or treatment assignment, the outcome, and confounders. [18, 19] This guides the conceptual thinking on whether certain variables are confounders, including measured and unmeasured confounders. Emulating the target trial requires that the necessary data elements are captured in the observational data source, as outlined in Table III.
For this example, we needed data on the diagnosis of stroke (population), type of inpatient stroke rehabilitation (treatment strategy), fracture risk (outcome), and a wide range of prognostic factors (confounders), in addition to other data elements for the eligibility criteria and lost to follow-up. This represents the necessary data requirement to execute target trial emulation. Therefore, when designing a comparative effectiveness study with observational data for target trial emulation, we need to map the observational data and analysis onto the specifications of the target trial. However, due to data constraints, we may need to modify the protocol components, such as eligibility criteria, treatment strategies, outcome, or follow-up period. We aim to design a target trial as close to the ideal trial as possible based on available observational data by outlining the protocol, describing how observational data is used to emulate the target trial, and explaining how the target trial differs from the ideal trial. [6, 23–25]
Regarding the analytic plan using observational data, we aim to examine the intention-to-treat effect, which compares the outcomes of groups assigned to each treatment at baseline, where possible based on the observational data available. [5, 6, 20, 21] This includes comparing the initiation of different treatments (e.g., data on the prescription of different drugs) as an observational analog of the intention-to-treat analysis. Baseline confounders are accounted for in the analysis in the absence of randomization to a treatment strategy. In our example, the analytic plan includes adjustment of baseline confounders/prognostic factors, and potential confounders of the association between service delivery of inpatient rehabilitation and risk of fracture include age, sex, previous fractures and health-related conditions, medication use, and stroke-specific factors including hemiplegia/hemiparesis, visual impairment, stroke type and severity, cognitive function, and functional status after stroke. [22, 26]
Use of observational data for target trial emulation
Table 4
|
Target trial emulation typically involves analysis of observational data from large databases, such as disease registries, population health surveys, administrative data, or potentially multiple data sources that are linked. This is because the observational data needs to capture information aligned with the eligibility criteria, treatment strategy, outcome, and a wide range of potential confounders to emulate the target trial. This is the necessary data requirement to execute target trial emulation for our example of inpatient stroke rehabilitation (Table IV provides an example of these data sources).
For example, if observational data sources do not measure the components of the rehabilitation program or the outcome of interest, we would not be able to emulate the target trial. Similarly, if the observational data sources do not capture information on a wide range of potential confounders, there is the risk of residual confounding from unmeasured confounders and the emulated study would not provide valid estimates of effects. If applicable, it is important to consider the feasibility of linking multiple databases if needed to conduct target trial emulation for the research at hand, as there may be ethical or administrative barriers to data linkages. Our target trial example focused on inpatient stroke rehabilitation proposes the use of multiple large registries and administrative databases that can be linked at ICES (originally known as the Institute for Clinical Evaluative Sciences) (Table IV).
Overall, the target trial emulation framework guides the design of comparative effectiveness studies using observational data to emulate a protocol that would be used for the target trial (randomized trial). In the example above, potential challenges to conducting this RCT on examining the effects of dedicated units of inpatient stroke rehabilitation on the risk of fracture include the use of rehabilitation process rolled out in different units over time, feasibility, and resources concerning 2–year follow-up for assessing the risk of fracture. To inform timely decisions on types of inpatient stroke rehabilitation, we can consider designing comparative effectiveness studies that emulate the target trial causal question and adapt the target trial protocol using observational data. Therefore, this framework can be used to apply principles of causal inference to estimate the effects of rehabilitation when a randomized trial cannot be conducted, such as because of feasibility, ethical reasons, or time/resource constraints.
Limitations of target trial emulation
To emulate randomization to the intervention versus comparison, we need to adjust for confounders to ensure comparability of the two groups at baseline. The selection of confounders should be informed by DAGs. [18, 19] Various methods can be used to control for confounders, including matching (e.g., propensity score matching), stratification or regression, standardization or inverse probability weighting, g-estimation, or doubly robust methods, as further described by Hernán and Robins. [6] It is not possible to emulate the target trial, particularly random assignment, if the observational data used does not measure a wide range of potential confounders or if we do not adjust for identified confounders in the analysis plan. Therefore, the observational data source(s) requires measurement of the treatment strategies, outcome (including any adverse effects if possible), and potential confounders.
Example of advanced analytic method to achieve
comparability of groups at baseline: propensity score matching
As an example, propensity score matching can be used to account for a wide range of potential confounders in aims to achieve comparability of two groups at baseline. The propensity score is the probability of treatment assignment (e.g., receiving treatment versus no treatment) conditional on observed baseline characteristics. [27] The propensity score is a balancing score, whereby conditional on the propensity score, the distribution of observed baseline covariates is similar between treatment groups (treated versus untreated). [27] This involves conducting a logistic regression model that includes the potential confounders as independent variables and treatment assignment (e.g., treated versus untreated) as the dependent variable to compute and output the propensity score. Subjects between the two treatment groups can be matched on this propensity score, and balance diagnostics assessed to determine whether there is sufficient balance in baseline covariates (<10% standardized mean difference). [28] The propensity-score matched cohort can then be used to assess for differences in the outcome(s). Details on propensity score methods can be found in Austin’s introduction to propensity score methods, [27] which provides other resources on these methods.
It is important to acknowledge that residual confounding from unmeasured confounders remains a limitation of a comparative effectiveness study using target trial emulation. Nevertheless, if there is unmeasured confounding (i.e., confounder not captured in the observational data source), methods can be used to estimate its potential impact, with various methods further described by Lash et al. [29, 30] and VanderWeele et al. [31, 32] As one approach, quantitative bias analyses can be conducted as a sensitivity analysis to assess the potential impact of residual confounding from unmeasured confounders.31, 32 Among various methods within quantitative bias analyses, an approach by VanderWeele et al. assesses how robust an association is to potential unmeasured confounding through a measure called the E-value. [32] E-value is defined as the minimum strength of association (on the risk ratio scale) that an unmeasured confounder would need to have with both the exposure and outcome to fully explain away the observed exposure-outcome association, conditional on measured covariates. [32] A large E-value suggests that considerable unmeasured confounding (i.e., an unmeasured confounder strongly associated with both the treatment and outcome) would be needed to explain away an effect estimate: [31] This allows researchers and others to consider how robust treatment-outcome associations are by assessing whether confounder associations of that magnitude are likely plausible. [32]
Calculation of E-value in quantitative bias analysis.
For an observed risk ratio (RR), the E-value calculation is: [32]
Table 5
|
E-value = RR + √[RR×(RR–1)].
The mathematical proof for this E-value formula is described elsewhere.33 This formula applies to an RR>1, so for RR<1, the inverse of the observed RR is used in the formula. See Table V for an example of calculating and interpreting an E-value.
Other limitations include that target trial emulation using observational data cannot be used to study new treatments not yet used in practice and not captured in observational data sources. Routine practice also does not use placebo/sham or blinding of outcome assessment; thus, studies using target trial emulation in this area emulate pragmatic RCTs of rehabilitation. This aligns with rehabilitation that tends to not have a valid placebo/sham due to the nature of the intervention.
Conclusions
This work describes the use of target trial emulation by Hernán and colleagues as applied to rehabilitation. This approach is important to obtain valid estimates of the causal effects of complex interventions, including rehabilitation, because there are circumstances when evidence needs to be generated rapidly, or when RCTs are not ethical or feasible. While there are limitations to this framework as described in this paper, target trial emulation serves as a methodologically robust option to RCTs. Overall, we aim to strengthen methodological approaches used to estimate causal effects of rehabilitation when RCTs cannot be conducted.
Conflicts of interest:
The authors certify that there is no conflict of interest with any financial organization regarding the material discussed in the manuscript.
Funding:
This study was supported and funded by the Italian Ministry of Health - Ricerca Corrente 2023. In addition, Pierre Côté’s contribution to the project was supported by the Canada Research Chairs Program and Jessica Wong was supported by a Banting Postdoctoral Fellowship from the Canadian Institute of Health Research. The funders had no role in study design, data collection and analysis, decision to publish, or preparation of the manuscript.
Participants in the 5th:
Christopher COLVIN, Claudio CORDANI, Anne CUSICK, Matteo J. DEL FURIA, Susanna EVERY-PALMER, Peter FEYS, Christoph GUTENBRUNNER, Carsten B. JUHL, William M. LEVACK, Wendy MACHALICEK, Rachelle MARTIN, Federico MERLO, Thorsten MEYER-FEIL, Luca MIRANDA, Bianca MOSCONI, Randolph NUDO, Aydan ORAL, and Cecilie RØE
References:
Dillen H, Bekkering G, Gijsbers S, Vande Weygaerde Y, Van Herck M, et al.
Clinical effectiveness of rehabilitation in ambulatory care for
patients with persisting symptoms after COVID-19:
a systematic review.
BMC Infect Dis 2023;23:419.
https://www.ncbi.nlm.nih.gov/entrez/query.fcgi?cmd=
Retrieve&db=PubMed&list_uids=37344767&dopt=Abstract
10.1186/s12879-023-08374-x
Metzl JD, McElheny K, Robinson JN, Scott DA, Sutton KM, Toresdahl BG.
Considerations for Return to Exercise Following Mild-to-Moderate
COVID-19 in the Recreational Athlete.
HSS J 2020;16(Suppl 1):102–7.
https://www.ncbi.nlm.nih.gov/entrez/query.fcgi?
cmd=Retrieve&db=PubMed&list_uids=32837412&dopt=Abstract
10.1007/s11420-020-09777-1
World Health Organization.
Living guidance for clinical management of COVID-19; 2021 [Internet].
Available from:
https://www.who.int/publications/i/item/WHO-2019-nCoV-
clinical-2021-2 [cited 2024, Jan 29].
National Clinical Guideline Centre (UK).
Stroke Rehabilitation: Long Term Rehabilitation After Stroke.
London: Royal College of Physicians (UK); 2013.
Hernán MA, Wang W, Leaf DE.
Target Trial Emulation:
A Framework for Causal Inference From Observational Data.
JAMA 2022;328:2446–7. https://www.ncbi.nlm.nih.gov/entrez/query.fcgi?
cmd=Retrieve&db=PubMed&list_uids=36508210&dopt=Abstract
10.1001/jama.2022.21383
Hernán MA, Robins JM.
Using Big Data to Emulate a Target Trial When
a Randomized Trial Is Not Available.
Am J Epidemiol 2016;183:758–64.
https://www.ncbi.nlm.nih.gov/entrez/query.fcgi?
cmd=Retrieve&db=PubMed&list_uids=26994063&dopt=Abstract
10.1093/aje/kwv254
Malmivaara A.
Benchmarking Controlled Trial—
a novel concept covering all observational effectiveness studies.
Ann Med 2015;47:332–40.
https://www.ncbi.nlm.nih.gov/entrez/query.fcgi?
cmd=Retrieve&db=PubMed&list_uids=25965700&dopt=Abstract
10.3109/07853890.2015.1027255
Nix HP, Weijer C.
Uses of equipoise in discussions of the ethics of randomized
controlled trials of COVID-19 therapies.
BMC Med Ethics 2021;22:143.
https://www.ncbi.nlm.nih.gov/entrez/query.fcgi?
cmd=Retrieve&db=PubMed&list_uids=34674679&dopt=Abstract
10.1186/s12910-021-00712-5
Negrini S, Kiekens C, Levack WM, Meyer-Feil T, Arienti C, Côté P;
Participants in the 5th Cochrane Rehabilitation Methodological Meeting.
Improving the quality of evidence production in rehabilitation.
Results of the 5th Cochrane Rehabilitation Methodological Meeting.
Eur J Phys Rehabil Med 2023.
https://www.ncbi.nlm.nih.gov/entrez/query.fcgi?
cmd=Retrieve&db=PubMed&list_uids=38112680&dopt=Abstract
10.23736/S1973-9087.23.08338-7
Alexander JH.
Equipoise in Clinical Trials:
Enough Uncertainty in Whose Opinion?
Circulation 2022;145:943–5.
https://www.ncbi.nlm.nih.gov/entrez/query.fcgi?
cmd=Retrieve&db=PubMed&list_uids=35344405&dopt=Abstract
10.1161/CIRCULATIONAHA.121.057201
Negrini S, Selb M, Kiekens C, Todhunter-Brown A, Arienti C, Stucki G, et al. ;
3 Cochrane Rehabilitation Methodology Meeting participants.
Rehabilitation Definition for Research Purposes:
A Global Stakeholders’ Initiative by Cochrane Rehabilitation.
Am J Phys Med Rehabil 2022;101:e100–7.
https://www.ncbi.nlm.nih.gov/entrez/query.fcgi?
cmd=Retrieve&db=PubMed&list_uids=35583514&dopt=Abstract
10.1097/PHM.0000000000002031
Heil TC, Verdaasdonk EG, Maas HA, van Munster BC, Rikkert MG, de Wilt JH, et al.
Improved Postoperative Outcomes after Prehabilitation for
Colorectal Cancer Surgery in Older Patients:
An Emulated Target Trial.
Ann Surg Oncol 2023;30:244–54.
https://www.ncbi.nlm.nih.gov/entrez/query.fcgi?
cmd=Retrieve&db=PubMed&list_uids=36197561&dopt=Abstract
10.1245/s10434-022-12623-9
Rubin DB.
Estimating causal effects of treatments in randomized
and nonrandomized studies.
J Educ Psychol 1974;66:688.
10.1037/h0037350
Hernán MA.
A definition of causal effect for epidemiological research. vJ Epidemiol Community Health 2004;58:265–71.
https://www.ncbi.nlm.nih.gov/entrez/query.fcgi?
cmd=Retrieve&db=PubMed&list_uids=15026432&dopt=Abstract
10.1136/jech.2002.006361
Hernan MA, Robins JM.
Causal Inference: What If.
Boca Raton, FL: Chapman & Hall/CRC; 2020.
Lash TL, VanderWeele TJ, Haneuse S, Rothman KJ.
Modern Epidemiology.
Wolters Kluwer; 2021.
Igelström E, Craig P, Lewsey J, Lynch J, Pearce A, Katikireddi SV.
Causal inference and effect estimation using observational data.
J Epidemiol Community Health 2022;76:960–6.
10.1136/jech-2022-219267
VanderWeele TJ, Hernán MA, Robins JM.
Causal directed acyclic graphs and the direction of
unmeasured confounding bias.
Epidemiology 2008;19:720–8.
https://www.ncbi.nlm.nih.gov/entrez/query.fcgi?
cmd=Retrieve&db=PubMed&list_uids=18633331&dopt=Abstract
10.1097/EDE.0b013e3181810e29
Lipsky AM, Greenland S.
Causal Directed Acyclic Graphs.
JAMA 2022;327:1083–4.
https://www.ncbi.nlm.nih.gov/entrez/query.fcgi?
cmd=Retrieve&db=PubMed&list_uids=35226050&dopt=Abstract
10.1001/jama.2022.1816
Hernán MA.
With great data comes great responsibility:
publishing comparative effectiveness research in epidemiology.
Epidemiology 2011;22:290–1.
https://www.ncbi.nlm.nih.gov/entrez/query.fcgi?
cmd=Retrieve&db=PubMed&list_uids=21464646&dopt=Abstract
10.1097/EDE.0b013e3182114039
Hernán MA.
Methods of Public Health Research -
Strengthening Causal Inference from Observational Data.
N Engl J Med 2021;385:1345–8.
https://www.ncbi.nlm.nih.gov/entrez/query.fcgi?
cmd=Retrieve&db=PubMed&list_uids=34596980&dopt=Abstract
10.1056/NEJMp2113319
Foley N, Meyer M, Salter K, Bayley M, Hall R, Liu Y, et al.
Inpatient stroke rehabilitation in Ontario:
are dedicated units better?
Int J Stroke 2013;8:430–5.
https://www.ncbi.nlm.nih.gov/entrez/query.fcgi?
cmd=Retrieve&db=PubMed&list_uids=22335859&dopt=Abstract
10.1111/j.1747-4949.2011.00748.x
Zhang Y, Thamer M, Kaufman J, Cotter D, Hernán MA.
Comparative effectiveness of two anemia management strategies
for complex elderly dialysis patients.
Med Care 2014;52(Suppl 3):S132–9.
https://www.ncbi.nlm.nih.gov/entrez/query.fcgi?
cmd=Retrieve&db=PubMed&list_uids=24561752&dopt=Abstract
10.1097/MLR.0b013e3182a53ca8
Garcia-Albeniz X, Chan JM, Paciorek A, Logan RW, Kenfield SA, Cooperberg MR, et al.
Immediate versus deferred initiation of androgen deprivation
therapy in prostate cancer patients with PSA-only relapse.
An observational follow-up study.
Eur J Cancer 2015;51:817–24. https://www.ncbi.nlm.nih.gov/entrez/query.fcgi?
cmd=Retrieve&db=PubMed&list_uids=25794605&dopt=Abstract
10.1016/j.ejca.2015.03.003
Zuo H, Yu L, Campbell SM, Yamamoto SS, Yuan Y.
The implementation of target trial emulation for causal inference:
a scoping review.
J Clin Epidemiol 2023;162:29–37.
https://www.ncbi.nlm.nih.gov/entrez/query.fcgi?
cmd=Retrieve&db=PubMed&list_uids=37562726&dopt=Abstract
10.1016/j.jclinepi.2023.08.003
Kapral MK, Fang J, Alibhai SM, Cram P, Cheung AM, Casaubon LK, et al.
Risk of fractures after stroke:
Results from the Ontario Stroke Registry.
Neurology 2017;88:57–64.
https://www.ncbi.nlm.nih.gov/entrez/query.fcgi?
cmd=Retrieve&db=PubMed&list_uids=27881629&dopt=Abstract
10.1212/WNL.0000000000003457
Austin PC.
An Introduction to Propensity Score Methods for Reducing
the Effects of Confounding in Observational Studies.
Multivariate Behav Res 2011;46:399–424.
https://www.ncbi.nlm.nih.gov/entrez/query.fcgi?
cmd=Retrieve&db=PubMed&list_uids=21818162&dopt=Abstract
10.1080/00273171.2011.568786
Austin PC.
Balance diagnostics for comparing the distribution of baseline covariates
between treatment groups in propensity-score matched samples.
Stat Med 2009;28:3083–107.
https://www.ncbi.nlm.nih.gov/entrez/query.fcgi?
cmd=Retrieve&db=PubMed&list_uids=19757444&dopt=Abstract
10.1002/sim.3697
Lash TL, Fox MP, Fink AK.
Applying quantitative bias analysis to epidemiologic data.
Springer Science & Business Media; 2011.
Lash TL, Fox MP, MacLehose RF, Maldonado G, McCandless LC, Greenland S.
Good practices for quantitative bias analysis.
Int J Epidemiol 2014;43:1969–85.
https://www.ncbi.nlm.nih.gov/entrez/query.fcgi?
cmd=Retrieve&db=PubMed&list_uids=25080530&dopt=Abstract
10.1093/ije/dyu149
Vanderweele TJ, Arah OA.
Bias formulas for sensitivity analysis of unmeasured
confounding for general outcomes, treatments, and confounders.
Epidemiology 2011;22:42–52.
https://www.ncbi.nlm.nih.gov/entrez/query.fcgi?
cmd=Retrieve&db=PubMed&list_uids=21052008&dopt=Abstract
10.1097/EDE.0b013e3181f74493
VanderWeele TJ, Ding P.
Sensitivity Analysis in Observational Research:
introducing the E-Value.
Ann Intern Med 2017;167:268–74.
https://www.ncbi.nlm.nih.gov/entrez/query.fcgi?
cmd=Retrieve&db=PubMed&list_uids=28693043&dopt=Abstract
10.7326/M16-2607
Ding P, VanderWeele TJ.
Sensitivity Analysis Without Assumptions.
Epidemiology 2016;27:368–77.
https://www.ncbi.nlm.nih.gov/entrez/query.fcgi?
cmd=Retrieve&db=PubMed&list_uids=26841057&dopt=Abstract
10.1097/EDE.0000000000000457
Return to REHABILITATION DIPLOMATE
Since 11-05-2024
|